Since the emergence of ChatGPT, the AI boom has lasted for two years. During this time, the general public has been thrilled by the capabilities of large language models, which can generate smooth and natural text from simple commands, turning science fiction scenarios into reality.
The field of large models is also entering a critical phase, where new technologies must be transformed into new products that meet real needs and develop into a new commercial ecosystem.
Just as mobile payments, smartphones, and LTE collectively fueled the prosperity of the mobile internet era, the AI industry is also seeking such a product-market fit (PMF) in 2024.
The era of exploring new technologies has begun, and whether a new frontier can be discovered will determine if large models are just another money-burning capital game, a replay of the internet bubble, or, as Jensen Huang said, the beginning of a new industrial revolution. This answer will be revealed faster than artificial general intelligence (AGI).
The Big Issues with Large Models
Today, the competition in foundational models has basically stabilized. Led by OpenAI, with ChatGPT firmly holding the market lead, other players like Anthropic, DeepMind, Llama, and Grok each have their strengths.
Thus, the most exciting thing in 2024 is not who has expanded parameters or improved response speed, but how large model technology can become a usable product.
From the start, applying large language model technology has been a challenge. Harvard Business Review conducted a survey and found that there are as many as 100 types of generative AI applications.
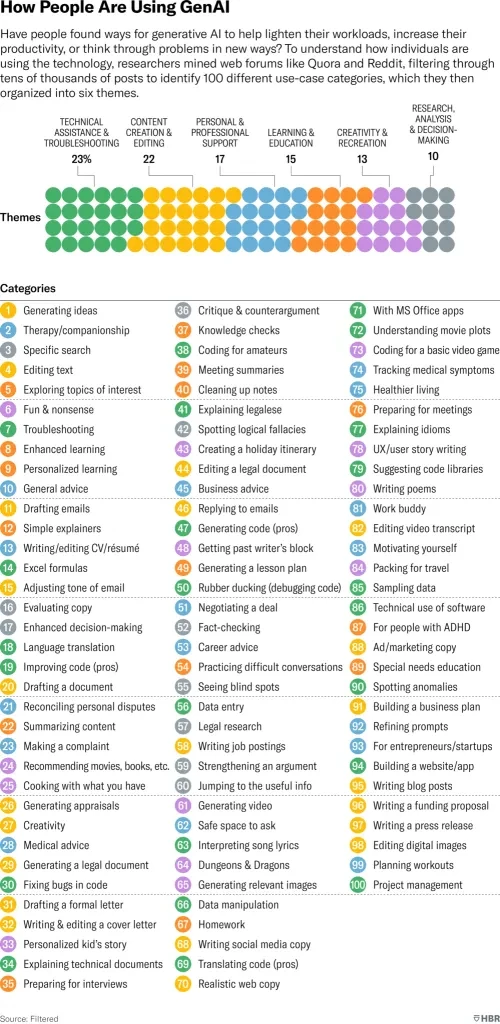
However, they fall into five main categories: technical problem-solving, content production and editing, customer support, learning and education, and artistic creation and research.
The well-known investment firm a16z has shared their team’s top generative AI products, including familiar names like Perplexity, Claude, and ChatGPT. There are also more niche products like note-taking apps Granola, Wispr Flow, Every Inc., and Cubby. In the education sector, 2024’s biggest winner was NotebookLM, along with chatbots like Character.ai and Replika.
For ordinary users, most of these products are free, and subscription or professional versions are not necessary expenses. Even for a strong player like ChatGPT, subscription revenue in 2024 was about $283 million per month, doubling from 2023. But in the face of huge costs, this income seems insignificant.
Enjoying technological advancements is a joy for ordinary users, but for industry professionals, no matter how exciting the technological evolution is, it cannot remain in the lab; it must enter the commercial world for testing. The subscription model has not been widely accepted, and the time for embedding ads has not yet arrived. The time left for large models to burn money is running out.
In contrast, business-oriented development is more promising.
Since 2018, the mention of AI in Fortune 500 earnings calls has nearly doubled. In all earnings calls, 19.7% of the records mention generative AI as the most discussed topic.
This is also the consensus of the entire industry. According to the “Artificial Intelligence Development Report (2024)” blue book released by the China Academy of Information and Communications Technology, by 2026, over 80% of enterprises will use generative AI APIs or deploy generative applications.
Applications for enterprises and consumers show different development trends: consumer-facing applications emphasize low barriers and creativity, while enterprise-facing applications focus more on professional customization and efficiency feedback.
In other words, improving efficiency is something every company pursues and wants to achieve, but just saying these four words is too vague. Large models need to prove they can genuinely solve problems in use cases and truly enhance efficiency.
Precisely Finding Entry Points to Implement Technology
Whether in terms of resource investment or market expansion efforts, China’s competition in large models was intense throughout 2024.
According to data from China’s Ministry of Industry and Information Technology, the growth rate of China’s large language model market in 2023 exceeded 100%, reaching approximately $2 billion. Companies are actively experimenting with commercialization, initially engaging in price wars: reducing costs through token-based billing, API calls, and other methods. Many mainstream large models are now nearly free.
Lowering prices and costs is relatively easy to achieve. However, understanding business and analyzing entry scenarios is a more challenging path.
Not all companies participate in price wars, relying on low-cost competition.
“In this situation, it’s more important to find our unique features and leverage our strengths. Tencent has many internal scenarios that provide us with more insights and further enhance our capabilities,” said Zhao Xinyu, AI Product Specialist at Tencent Cloud and Head of Tencent Hunyuan ToB Products. “Externally, we focus on one industry, concentrate on specific scenarios within that industry, and then gradually expand.”
Among many foundational models, Hunyuan may not be the most attention-grabbing, but its technical strength is undeniable.
In September 2023, Hunyuan released the general text-to-text model Hunyuan Turbo, adopting a new Mixture of Experts (MoE) structure. It performed well in language understanding and generation, logical reasoning, intent recognition, as well as coding, long-context, and aggregation tasks. In the dynamic update version of November 2023, it was upgraded to the best-performing model across the board. Currently, Tencent Hunyuan’s capabilities are being fully delivered through Tencent Cloud, offering various sizes and types of models, combined with other AI products and capabilities from Tencent Cloud Intelligence, to help model applications land in scenarios.
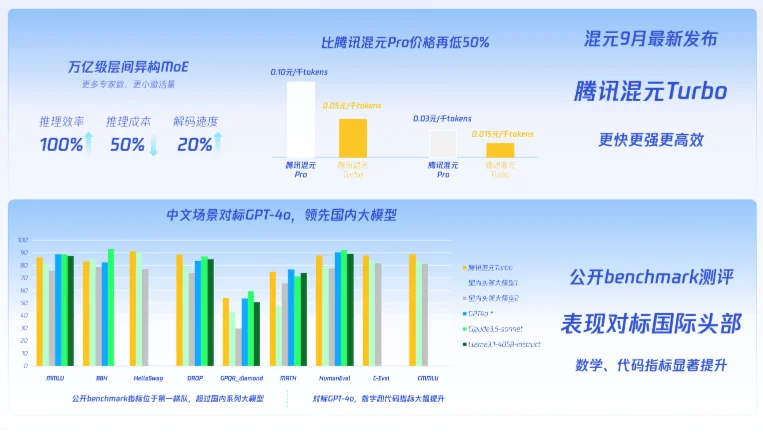
Currently, the application forms of models are roughly divided into two types: serious scenarios and entertainment scenarios. The latter includes chatbots, companion apps, etc.
“Serious scenarios” refer to applications in core business operations of enterprises, where accuracy and reliability are highly demanded. In these scenarios, large models need to handle structured information, usually following preset business processes and quality standards, and their application effects are directly related to the operational efficiency and business outcomes of enterprises.
Tencent Cloud once helped an outbound service provider build a customer service system, which is a typical serious scenario. Outbound calls involve natural language dialogue capabilities, content understanding, and analysis capabilities, which are highly compatible with large language models.
In fact, the challenge lies in the details. At that time, the team faced two core challenges. One was performance issues, as the model’s parameter size was huge, reaching 70B or 300B scale, how to complete the response within 500 milliseconds and pass it to the downstream TTS system became an important technical challenge.
The second was the accuracy of dialogue logic. The model would sometimes produce illogical responses in some dialogues, affecting the overall dialogue effect. To overcome these challenges, the project team adopted an intensive iteration strategy, maintaining a rapid iteration pace of one version per week within a 1-2 month development cycle.
Enterprise customers show interest in large language model technology and are willing to try innovations, but there is always a cognitive gap in the deep integration of technology and business. This does not stem from a lack of understanding of their own business by enterprises, but requires a professional technical team to deeply understand industry pain points and business scenarios, find the most suitable scenarios, tailor AI landing solutions for enterprises, and achieve the best combination of technology and business.
“The traditional approach might require operators to build (corpora) one scenario at a time,” Xinyu explained, “but with large models, you only need to give a prompt to meet the demand.” After clarifying the demand, the Hunyuan team almost updated a version every week, accelerating the iteration speed, and within one or two months, the accuracy reached 95%.
For this outbound service provider, generative technology was completely new. Hunyuan directly showed them the benefits brought by large models, reducing manpower expenses by three-quarters.
“The best approach is to showcase the effects,” Xinyu said. When customers have some understanding of generative technology but not much, showcasing the effects is the most effective. By finding scenarios that can be entered through the customer’s business experience, directly conducting test verification, and demonstrating the improvements that can be achieved.
A similar experience occurred in cooperation with Xiaomi, which was described as a “two-way journey.”
The other party wanted to introduce large models into Q&A interactions, applying AI search capabilities to terminals. This hit two of Hunyuan’s strengths: support provided by Tencent’s rich content ecosystem and Hunyuan’s capabilities in AI search. For Q&A, accuracy is very critical.
“There were still many difficulties at the beginning,” Xinyu recalled. “From their perspective, the business form covered multiple scenarios, including casual chat, knowledge Q&A, and other types, among which the knowledge Q&A scenario had relatively high accuracy requirements.”
Through preliminary testing, the Hunyuan team clarified their advantages in search scenarios, and together with the other party, gradually refined the broadly defined Q&A interaction according to different topic levels. This kind of segmentation allows the model to more clearly understand the specific needs and effect requirements of each scenario, thereby conducting more targeted optimization.
The knowledge Q&A scenario became the landing point. In subsequent implementations, Hunyuan still had many challenges to overcome: latency issues need not be mentioned, response time must be fast; secondly, the integration of search content.
“In the entire link, we built a self-developed search engine and an intent classification model to determine whether it is a high-timeliness question. For example, whether it is related to news or current affairs topics, and then decide whether to give it to the main model or AI search.”
Only calling the most needed parts greatly improves response speed. An important discovery is that 70% of inquiries lead to AI search, which means there must be sufficiently rich content as the most basic call support.
Behind Hunyuan lies the entire Tencent content ecosystem. From news, music, finance, to even healthcare, Tencent’s ecosystem offers a wealth of high-quality content. This content is accessible and referable by the Hunyuan model during searches, providing a unique advantage.
After more than two months of intensive iteration, the quality of responses, response speed, and performance have fully met the requirements and have been implemented in Xiaomi’s actual business operations.
The key to B2B business is generating revenue and gaining trust, which requires delivering real value to clients’ operations.
“Roll” Generalization to Reach More Scenarios
The application of large models across various industries and products is also fostering the growth of the technology itself.
For some large model products, choosing the B2C path involves a core consideration: using consumer feedback to optimize the model. The need for tuning large models is endless, and the number and activity of consumer users provide the fuel for model iteration, thereby accelerating the iteration speed.
In fact, this is also achieved in B2B business, with even higher demands.
The K12 Chinese essay grading feature of “Teenager Gains” utilizes Hunyuan’s multimodal capabilities. Combined with Tencent Cloud’s intelligent OCR technology, it recognizes students’ essay content and uses the large model to grade the essays based on preset scoring standards.
Typically, if the score difference between the large model and a human teacher is within five points, it’s considered good—but this is not easy to achieve. Initially, only 80% of Hunyuan’s scores were within five points of human teachers’ scores.
“The model has certain methods and capabilities to solve problems in some scenarios. However, when focusing on a specific client’s business, higher performance is required,” said Xinyu. “While 90% accuracy might meet business goals, at 70% or 80%, there’s still a gap.”
This means continued effort is needed. As the enterprise client base expands, new demands are placed on the technology itself: firstly, a significant increase in iteration speed—iterations for consumer users might take one to two months, but now a new version can appear weekly. This high-frequency iteration greatly promotes model growth and progress.
Secondly, continuously serving different enterprise scenarios has significantly enhanced the model’s generalization ability. This indicates that deeply serving diverse enterprise needs not only accelerates the pace of model development and iteration but also improves the model’s practicality and adaptability, allowing it to expand from serious scenarios to more entertainment-oriented ones.
The role-playing content platform “Dream Dimension,” which recently secured tens of millions in Series A funding, has applied the Hunyuan large model’s role-playing exclusive model, Hunyuan-role, aimed at serving young users. It combines generative AI technology to provide an interactive, story-driven virtual character interaction experience.
Hunyuan-role has pioneered a new form of human-computer interaction. By creating diverse virtual character images and based on preset story backgrounds and character settings, it engages users in natural and smooth interactive dialogues.
On the technical level, such scenario applications have demonstrated Hunyuan-role’s leading advantages in handling long and short text dialogues, intent recognition, and response. It can handle diverse application scenarios and exhibits excellent content humanization capabilities—not only engaging in warm dialogue interactions but also advancing storylines to create an immersive user experience.
These features make Hunyuan-role a powerful tool for product customer acquisition and user operations, playing a crucial role in enhancing user retention and engagement. It also reflects that Hunyuan, honed and improved in serious scenarios, has developed generalization capabilities that can cover broader scenarios, even in end-side applications.
Expanding from serious scenarios to entertainment, creativity, and more is a journey that large model applications must undertake.
As technology matures and costs decrease, large models are bound to expand into broader application scenarios. Initially focused on serious business scenarios like corporate office work, data analysis, and scientific research, these areas have clear demands and a higher willingness to pay.
Further expansion into entertainment, creativity, and content production requires a strategic anchor: always focusing on solving specific scenario needs as the core goal, pinpointing the entry point for integrating large model capabilities.
In addition to collaborating with application software, partnerships with hardware manufacturers are also needed to allow the model to perform and function on the consumer-facing end, providing services that are closer to users’ daily lives and offering more convenient, immediate service experiences.
In this process, market awareness and acceptance of generative AI technology are continuously increasing, and the user base is steadily expanding. In the face of this rapidly changing market environment, the model’s iteration capability becomes particularly important. This is not only reflected in technical performance but also in understanding user needs, adapting to different scenarios, and more. Only models and teams that can quickly learn, continuously optimize, and constantly adapt to new demands can maintain an advantage in the competition.
As more scenarios are continuously covered, the reach to more end consumers also expands. With the market’s overall acceptance of generative technology, the potential user base will continue to grow. A model that can quickly iterate and self-improve can keenly adapt to changes, moving more steadily and further.
Source from ifanr
Disclaimer: The information set forth above is provided by ifanr.com, independently of Alibaba.com. Alibaba.com makes no representation and warranties as to the quality and reliability of the seller and products. Alibaba.com expressly disclaims any liability for breaches pertaining to the copyright of content.