Today’s consumers are more demanding, expecting better products and experiences. If not satisfied, they often look elsewhere. A survey of 25,000 global consumers revealed that 64% want companies to respond more quickly to their evolving needs.
The traditional “make it, sell it, repeat” approach no longer meets customers’ expectations. Businesses must now anticipate what customers want before they know and deliver on those desires ahead of the competition.
But how exactly can businesses predict what their customers want? Continue reading as we explore 5 demand forecasting techniques to help customers feel heard and understood.
Table of Contents
What is demand forecasting and why is it important?
3 types of demand forecasting you need to know
5 demand forecasting techniques to help you avoid surprises
Real-time demand forecasting will revolutionize supply chains
What is demand forecasting and why is it important?
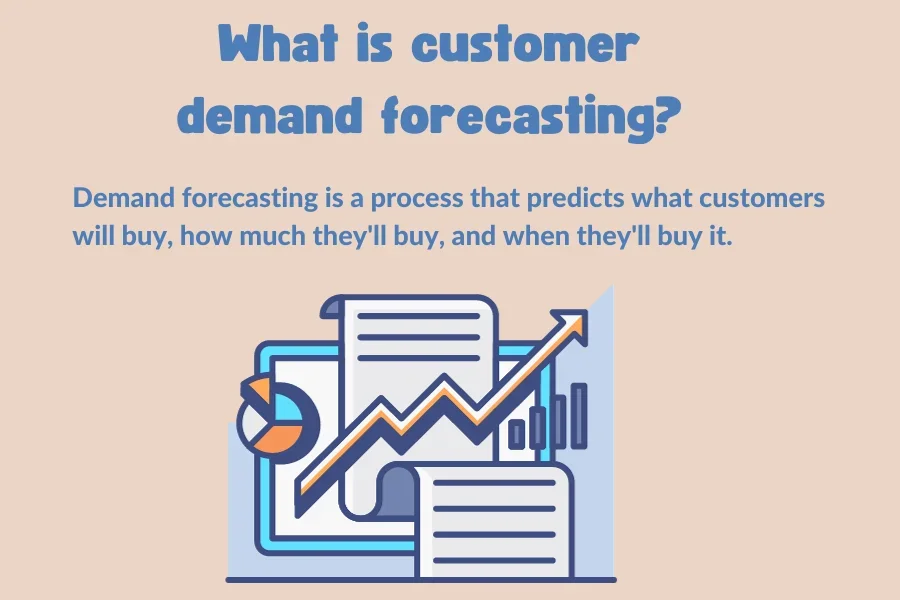
Demand forecasting is akin to being a meteorologist, but rather than predicting sunshine or rain, businesses forecast how much of their product customers will desire in the forthcoming periods. In other words, predicting customer demand is simply an estimation process determining future demand for a firm’s goods or services.
Such a prediction is not an outcome of guesswork. Instead, businesses use quantitative methods, such as statistical and econometric tools, and qualitative techniques, such as market surveys or expert opinion, to accurately predict demand trends. We will explore these methods more deeply in the following sections.
Demand forecasting is an important component of supply chain management, and companies can benefit tremendously from implementing it in various ways:
- Optimizing inventory management: By predicting future demand accurately, businesses can maintain optimal inventory levels. This can help them steer clear of stockouts, which could lead to lost sales and annoyed customers, or unnecessary overstocking, which ties up capital and creates storage issues.
- Reducing cost: Businesses can efficiently allocate resources by accurately predicting future demand and expanding or contracting production capacity and workforce. This can help reduce waste in resource allocation, reducing costs and improving profitability.
- Enhancing customer satisfaction: Understanding customer demand involves meeting or surpassing customer needs by ensuring the right products are available when and where they’re needed. Demand forecasts enable businesses to align their supply chains with customer demand, resulting in satisfied customers and wise business decisions.
3 types of demand forecasting you need to know
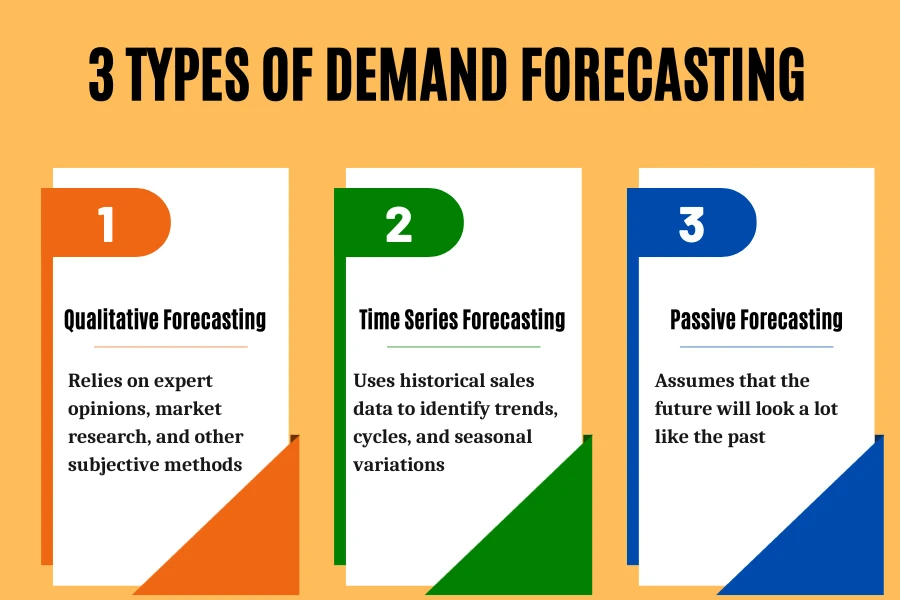
Demand forecasting involves a variety of tools and techniques. However, before we dive into these, it’s crucial that we first grasp the concept of demand analysis. Ready for a quick tour? Let’s explore the three primary types of demand forecasting:
Qualitative forecasting
Qualitative forecasting methods are usually used when historical data is unavailable or a business is launching a new product or entering a new market. It relies on the knowledge and experience of industry experts, stakeholder opinions, and other subjective factors rather than pure mathematical data.
For instance, let’s say a smartphone company is planning to launch a new phone model. They might use qualitative forecasting methods such as focus groups, surveys, or interviews to understand what features consumers might like, how much they’d be willing to pay, and how many units the company could expect to sell. The downside of qualitative forecasting? It is subjective and can be influenced by cognitive biases.
Time series demand forecasting
Time series demand forecasting is a quantitative forecasting approach to predicting future customer needs. This data-based method considers historical data to estimate future demand.
This includes assessing past sales data to identify patterns, growing trends, or any changes linked to different times of the year, which could influence future demand. It uses statistical methods such as linear regression and moving averages to build these demand forecasts.
Among these techniques, linear regression is like drawing the best possible straight line through a group of points on a graph. This line is the best guess of the underlying trend in the data, thus assisting in predicting future scenarios. If we see the past sales line trending upwards, it’s a useful indicator that future sales are also likely to increase.
Passive demand forecasting
Passive demand forecasting is another quantitative method that uses past sales data to predict future demand. But unlike time series demand forecasting, passive demand forecasting is considered more simplistic. It is typically ideal for businesses with strictly seasonal or steadily growing sales (companies that see the same pattern in sales year after year).
Let’s consider a small store that sells Christmas decorations. Like clockwork, their sales shoot up in November and December every year and are quiet for the rest of the year. This store could use passive demand forecasting by looking at their last November and December sales to get a good idea of what to expect this year. Using a simplistic example, if they sold 100 Christmas tree ornaments last December, and the business is growing steadily, they might expect to sell around 110 or 120 this year.
5 demand forecasting techniques to help you avoid surprises
Armed with knowledge of the various types of demand forecasting, it’s now time to explore the top five forecasting techniques in-depth and understand their nitty-gritty workings:
Delphi method
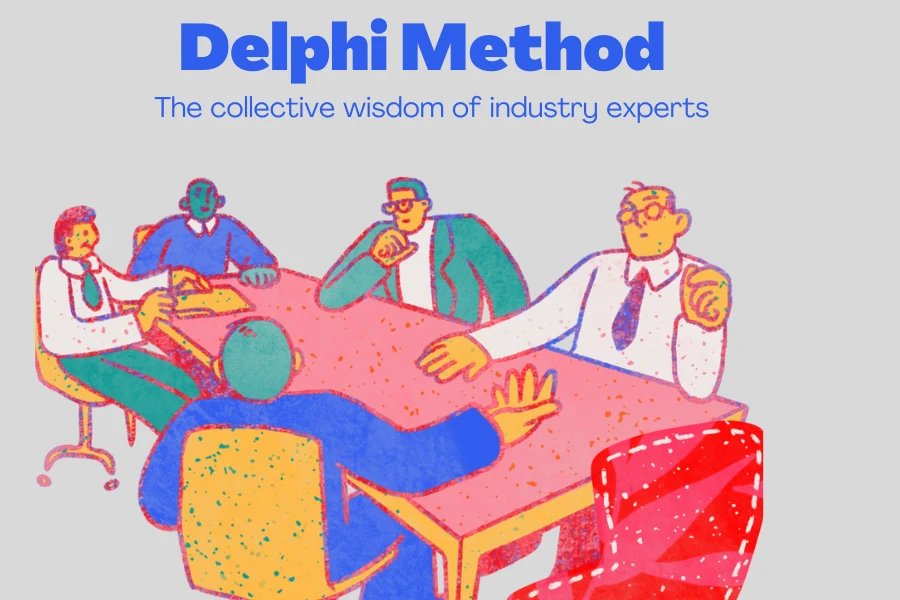
The Delphi method is an iterative qualitative process that leverages the collective wisdom of a group of experts. This iterative approach revolves around refining opinions and recommendations from various knowledgeable individuals to generate precise projections concerning a product or service’s future demand. Here is a breakdown of this iterative process:
- Selecting the experts: Assemble a diverse group of experts in the business field, including industry specialists, market analysts, or even internally experienced members of the organization with extensive market knowledge.
- Asking the question: The facilitator submits a survey to the experts, featuring queries relevant to the business’s future demand, such as, “What factors will influence our product demand in the next two years?”
- Gathering opinions: Survey responses are collected independently from each expert, ensuring a free expression of thoughts without interfering with others’ opinions.
- Sharing feedback: The facilitator compiles an overview of the key ideas and opinions conveyed in the responses and redistributes this summary to the group, allowing experts to examine general trends and perspectives from their peers.
- Reassessing opinions: With this new information, experts reassess their previous answers and make revisions based on insights gained from other participants.
- Repeating the process: To reach a consensus, the cycle of questioning, sharing feedback, and revising opinions is repeated until the experts’ predictions align.
Market research

Market research is a systematic process of gathering, analyzing, and interpreting valuable data about target consumers’ preferences, needs, and behavior. As a qualitative demand forecasting technique, it utilizes focus group discussions and surveys to understand consumer preferences and anticipate future demand for products or services.
Let’s consider a scenario where a brand sells delicious chocolate bars. They have noticed a steady decline in their traditional milk chocolate bar sales. Instead of panicking, they employed their best detective skills and conducted qualitative market research.
The brand organized virtual focus group discussions with chocolate lovers from different age groups, backgrounds, and geographic locations. They asked them about their chocolate preferences – Do they enjoy milk chocolate, or are they more into dark or white chocolate? Do they seek out single-origin chocolates? Do they prefer added nuts, fruits, or other ingredients within the chocolate bars?
They also sent out online surveys to a larger audience via their social media pages to gather further details on why they’re switching. Are they more health-conscious? Vegan? Or are they simply following Instagram trends?
Once the results were in, they found a growing demand for vegan chocolate, with consumers looking for alternative, dairy-free treats. They also found that their customers crave creativity – eager for exciting ingredients and unexpected flavor profiles.
Based on these insights, the brand launched a vegan-friendly dark chocolate bar with an exciting mix of sea salt and caramel. This isn’t a random decision based on whims; it’s strategic, calculated, and informed by shoppers’ preferences – all thanks to thorough market research.
Naïve forecasting model
The Naïve forecasting model is a straightforward quantitative method using the most recent period’s actual demand to determine the subsequent forecast. This model operates on the principle that history often repeats itself, indicating that past data can accurately predict future demand. Because it does not necessitate complicated computations or in-depth statistical insights, it is aptly termed ‘naïve.’
Suppose a business owner operates an e-commerce store specializing in eco-friendly reusable water bottles. These bottles have gained considerable popularity due to their environmentally friendly nature, exhibiting consistent demand over several months.
Utilizing the naïve forecasting model, the store owner would rely on the most recent month’s sales (e.g., 500 water bottles) to predict the demand for the upcoming month. Since the demand for reusable water bottles remains relatively stable without being affected by seasonal changes or market trends, the naïve model’s simplicity accurately forecasts the anticipated sales in the following month.
Probabilistic model
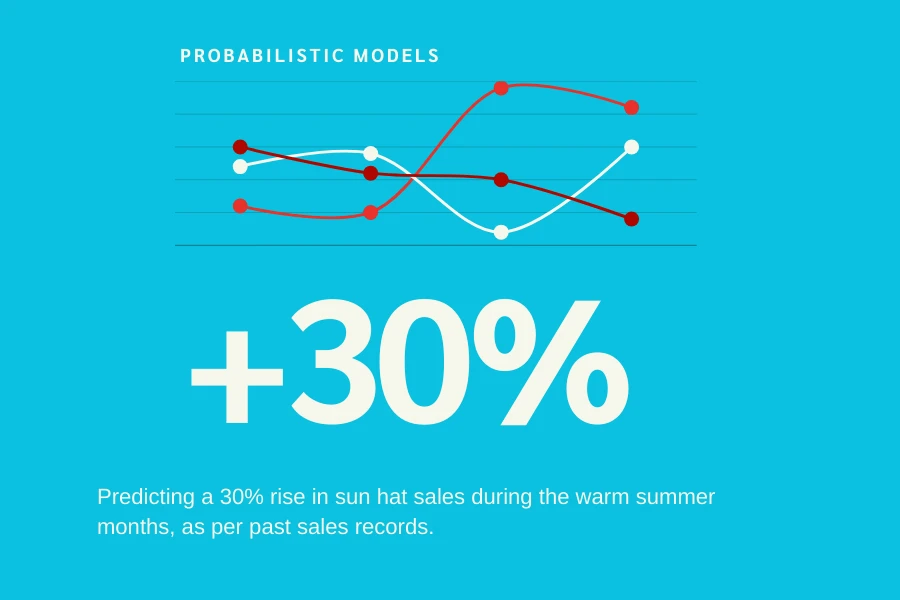
Probabilistic models are advanced statistical forecasting tools that rely heavily on mathematical formulas and statistical measures. They are used when the future is uncertain. ‘Probabilistic’ comes from the word ‘Probability,’ the mathematical term for the likelihood of an event occurring.
In the probabilistic model, businesses use past sales data and mathematical formulas to predict possible future demands. What does that mean? Simply, it’s like looking at past sales of sun hats in the summer months. If high sales happen every June, July, and August, a probabilistic model would also predict high sales in these months for the coming years based on the pattern it sees.
The “Poisson distribution” and the “Bayesian probability model” are popular probabilistic models:
Poisson model
Think of Poisson distribution as predicting rain. We might know that it’s likely to rain 10 days out of 30, but we can’t predict which specific days those will be — that’s random. Similarly, the Poisson distribution helps businesses estimate how many customers, say, are likely to pop into their store or website each day.
When using the Poisson distribution, a business owner can notice they sell about 100 hats daily on average. On sunny days, this number goes up, and on cloudy days, it sinks. Through the Poisson model, the shop can find out:
- The odds of selling, say 50 hats on a cloudy day,
- Or the high-flying 150 hats on a sunny day!
Bayesian model
The Bayesian probability model is about updating predictions as new data comes in. Let’s assume a garment brand is selling winter coats online. Based on historical data (prior beliefs), it’s observed that sales for winter coats in September have been relatively low. Thus, the brand might initially predict that this pattern will continue in the upcoming September season.
However, in the current September season, they see a sudden spike in sales midway through the month (new evidence). This data prompts them to update their initial prediction. One possible reason could be a sudden drop in temperature or an unusually early winter this year.
With this new data, the brand now adjusts its predictions using the Bayesian model, anticipating increased demand in the current and possibly the upcoming September season (posterior probability). The eCommerce business can stock up accordingly, ready to meet the unexpected demand.
Machine learning model
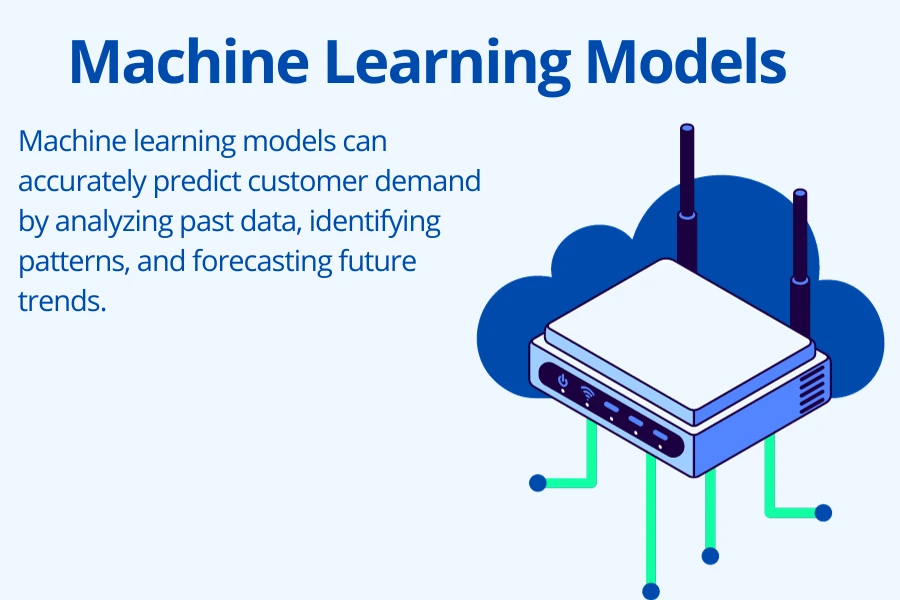
A machine learning model utilizes deep learning and neural networks to discern complex relationships. These relationships are often too intricate and non-linear for traditional statistical tools to unravel. Sounds impressive, doesn’t it?
Visualize it as a digital brain that ceaselessly learns from the patterns it observes, where, in this context, the patterns are consumer purchasing habits. Like how our brains reinforce memory through repetition and observation, machine learning models also adapt and refine understanding over time.
As an example, consider a business selling women’s beauty care products. They could leverage a tool like Alibaba’s AI solution. This platform simulates and examines market demand and then preprocesses the data using data intelligence and time series forecasting algorithms.
The machine learning model can identify emerging trends by analyzing customers’ purchasing patterns. For instance, it may notice a surge in the demand for sunscreen every summer. As another example, the model might detect that special edition lipsticks are highly sought after whenever the business initiates a new collaboration with a prominent makeup artist influencer.
Real-time demand forecasting will revolutionize supply chains
Whether businesses decide to use a qualitative method such as market research and surveys, or an advanced quantitative method like machine learning models (or a combination of both), it’s clear that demand forecasting is undergoing significant changes.
The evolution from periodic to real-time forecasting is driven by an increasing availability of instantaneous data about customer behavior. This immediacy means that businesses no longer need to wait weeks or months to gather data and make forecasts.
They can adjust quickly, allowing for more accurate decision-making. By doing this, businesses are able to respond more promptly to shifts in demand, leading to the creation of a more efficient and responsive supply chain. For more strategies and techniques on how to understand customers and their behaviors, check out the blog center!
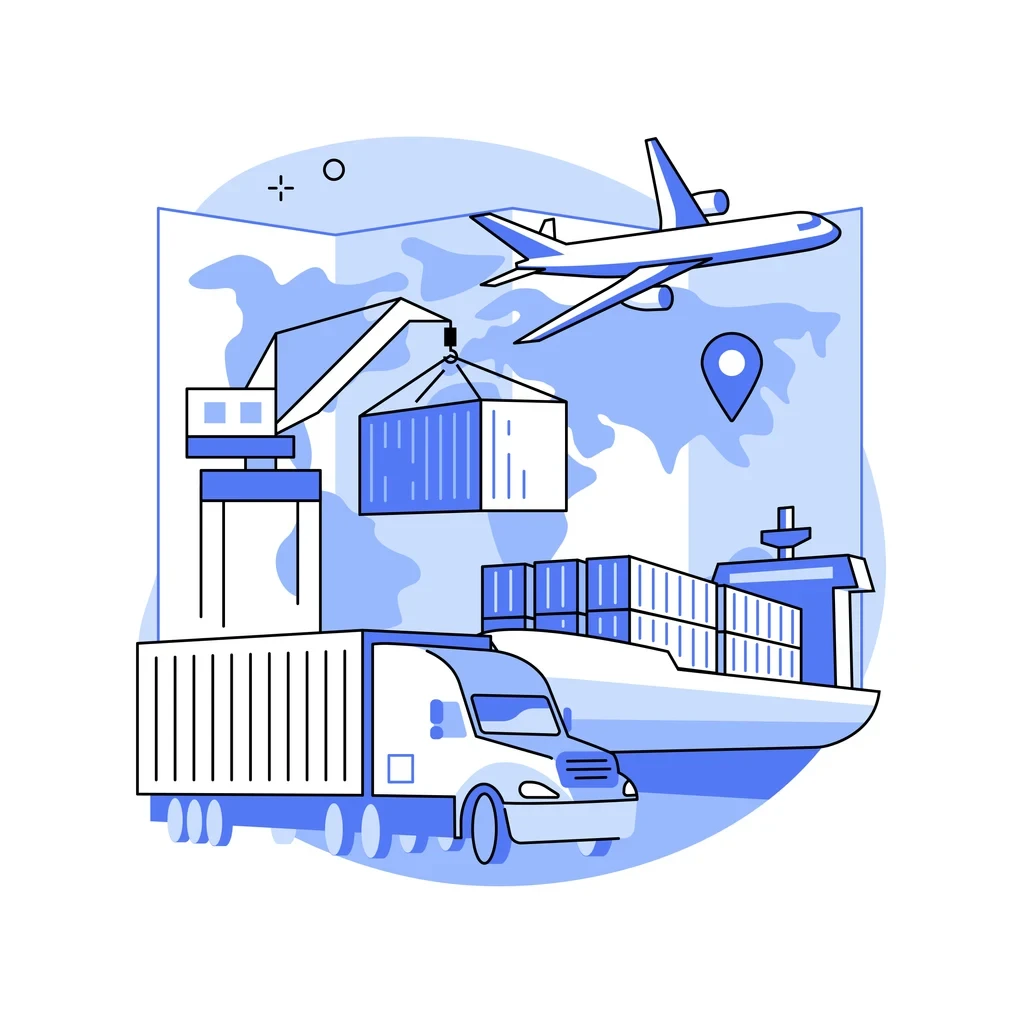
Looking for a logistics solution with competitive pricing, full visibility, and readily accessible customer support? Check out the Alibaba.com Logistics Marketplace today.